Predicting the Future: Driving Fundraising Outcomes with Models
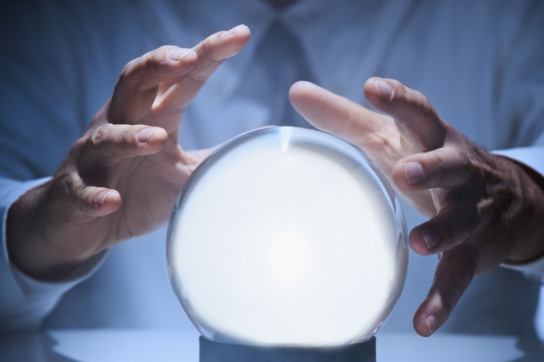
My first experience with modeling happened at the University of Washington development office in the fall of 1992. I arrived as the freshly-minted director of prospect research. Life was primitive back then. Real people did not have cell phones, and the cell phones that existed were the size of bricks. The Internet barely existed; we navigated it with tools like Gopher and TelNet. This was the first job I had that came with an email account. I was soon to learn all the lessons about what not to do by email the hard way.
Prior to my arrival, the development office had purchased a screening of the entire constituent database that provided two scores. One was a likelihood to give score, and the other was a wealth score. We also got some Claritas Prizm scores thrown in. Prizm is still around—it’s a lifestyle indicator that classifies people into groups with common characteristics. The groups had entertaining names like “Gold Coast,” “Gray Power,” and “Big Fish—Small Pond.” My favorite was “Shotguns & Pickups,” because it was so evocative of a specific lifestyle that I had some familiarity with.
My job, as the director of research, was to use the modeling results for prospecting—but also to sell them to the directors of development in the schools and colleges. I had a simple plan of attack:
- Cut the entire database into lists by school or college
- Take those lists to the director of development in each department
- Show them the top prospects identified by the screening
- Wait for the outpouring of gratitude
In fact, I typically got one or more of these reactions:
- “I already know all these people; tell me something I don’t know.”
- “I already know these people and they will never make a gift because they hate us.”
- “I already know these people and they aren’t wealthy enough to make a major gift.”
- (And this is the one that really drove me crazy) “I’ve never heard of these people. Why should I reach out to someone I don’t know?”
Gratitude was never once evidenced. In fact, most reactions resembled pity: “Poor naïve researcher, trying to mess up our fundraising expertise with science.”
I was too green and inexperienced to push back on the last reaction—the “who are these people” reaction. Today, it’s widely understood that this is why we use models: to find the unknown prospect—the whale swimming below the surface. We can use what we know about the people who do make big gifts to create models that identify those we never thought of as major gift prospects, but who match our model.
I will confess that this early experience with models made me a non-believer for quite a while. I became a champion of public data wealth screening. This takes what the prospect researcher does every day and soups it up to append public data to more records faster than ever before. It shifts the emphasis from reactive research to proactive by sorting your wealth screening results to reveal unexpected wealth. It’s an alternative way to spot the whales, and it’s a good one.
Wealth screening has its own set of problems, though. Just because someone is wealthy, does not mean they are going to give you a gift. And then there’s that annoying problem of privacy and respectful donor stewardship. Most wealth is private. I’ve handed profiles to major gift officers who threw them down in disgust, telling me that they know for a fact that this prospect has way more wealth than the profile reflects. Well, their inherited wealth, their private investments, their valuable collections—all private information. No researcher can put a precise value on any of that.
Over the years, I’ve learned that neither models nor wealth screening will save fundraising for nonprofits. In fact, I believe that they work very well hand in hand when there’s money in the budget and you have the staff and the expertise to make use of both. Sometimes you must make choices. And when you have to make choices, I’ve come full circle. A well-designed modeling project can do more to provide structure and direction to a fundraising program than can wealth screening alone.
What does it mean to have a well-designed project? To preface, I am not a statistician. I don’t make models. But after 14 years of delivering and selling them, I’ve learned a few things about what it takes to make good models—things that will make any statistical analysis better. Without these fundamentals, you could end up completely off the rails.
- Garbage in, garbage out. You need accurate demographic data about your prospects in order to make valid models. Get the deceased people out of the database. Clean up those addresses, make them as current as possible. As much as possible, identify which of the several addresses you might have for the constituent is the home address. That is the most relevant address to use in your modeling. The presence of other addresses might turn out to be useful, but nothing beats the home address for what it tells you about the life and situation of your prospect.
- Don’t assume you know what will turn out to be predictive. You may know in your gut that alumni who were part of the Greek system tend to be good donors, but it may turn out to be something else, or a cluster of other somethings, that becomes the key to predicting giving. Let go of your pet variables and let the statistics speak for themselves.
- Watch out for endogenous variables – the variables that are in some way the cause or the result of the thing you’re trying to predict. Endogenous variables always have a strong correlation to your dependent variable, but they are not necessarily predictive. You’re making a model for a women’s college and it turns out that gender is predictive? Imagine that! You might find that knowing someone’s email address is correlated with giving. Is it also possible that people who give are more likely to share their email with you?
- Correlation is not always causation. If you find that most of your donors were born before 1965, that might be predictive, but not if most of your constituent population is of a similar vintage. Your model can’t just show what your best donors have in common. It must show what makes them different from those who were given the opportunity to give but didn’t.
- Models require a good historical record. If you only have one year of giving data, you can’t predict who is likely to give next year. What separates the one-and-done donor from the truly committed supporter? Without a few years of consistent solicitations and donations, you just don’t have the basis for a strong model.
- As much as possible, append data to your file that comes from the outside. Demographic data, wealth data, philanthropic data, consumer marketing data. These additional data points broaden your view beyond what you have in your own database and may reveal correlations and predictive variables that make your models stronger than they would be if you remain limited to the data you have collected internally.
- Have a plan to use the results. You can create the most perfect model ever devised, but if it doesn’t change something about the way fundraising happens at your organization, it’s just a waste of time and resources. Too many modeling projects have foundered on the shoals of fundraiser indifference. Get buy-in from key stakeholders before you begin and get a commitment to use the results. It really does take a leap of faith for some development officers to reach out to people they don’t know. But the proof of the pudding is in the tasting. Tasting must happen.
Ultimately, a good model is not a crystal ball foretelling the future with precision. It isn’t a guarantee that constituents will behave one way or the other. But a good model is a way to reduce uncertainty. If you pick any constituent at random, you will be highly uncertain that the person will perform any particular action, such as making a gift. But a good predictive model will provide a better filter to highlight those most likely to give or upgrade their gift. You reduce your uncertainty about the outcome of your fundraising by giving your best attention to the highest scoring people. It’s true that not every high scoring person will ultimately do the thing you’re asking for, and some with low scores will do it, even with very little encouragement. But we know that most people give because they are asked to do so. By giving your best effort to those identified by the model, you improve your chances of getting to “yes.”