Making Sense of the Buzz Around ChatGPT
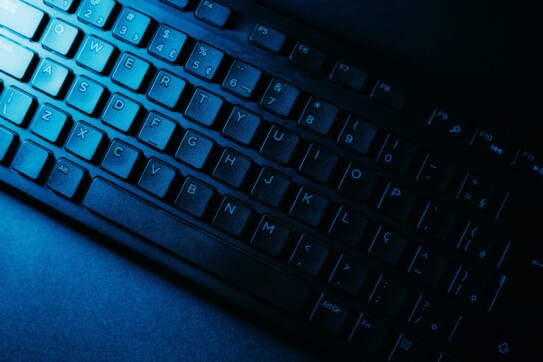
The Analytics team at Blackbaud has been playing around with ChatGPT over the past few months and we thought it might be helpful to share our observations. The initial wave of generative-AI examples in the art, music, and written composition spaces has generated a lot of interest within the technology sector. The second wave, however—coming after the launch of Google’s Bard and Microsoft Bing—has resonated more broadly and has implications and potential applications for nonprofit organizations.
Your charitable organization has probably fielded questions from curious supporters and internal audiences on the ways it might be leveraged. Is it just a fad? Or is generative AI the Napster of the 2020s, a pivotal, transformational moment that could signal the beginning of a fast, strange upswing in the next technological sigmoid curve?
For this post we’ll highlight a few examples from our own experimentation, explaining what we see going on and what we’ve learned. We’ll summarize a few key takeaways and point to some resources that might be helpful as you think through the impact of this technology. Finally, we’ll identify some viable use cases for your organization.
How ChatGPT Works
ChatGPT is an artificial intelligence model that generates content (generative AI) based on an amalgamation of secondary sources; early versions used written communication as a training dataset for the model and predicted the most likely next word based on the input data provided to the model.
A few key things to keep in mind:
- AI measures probabilistic outcomes in response to a question to determine what data it should show. Basically: answer A is 56% likely; B is 23% likely, etc.
- ChatGPT is a probabilistic model. It has no understanding of the content; only what word is most likely to come next.
- ChatGPT’s real value lies in its ability to reduce uncertainty in ways that introduce system-wide change, prompting creativity as we reimagine old ways of doing things.
So what, exactly, is so compelling about generative AI or ChatGPT? Perhaps it has something to do with the personality or styling of responses that make it almost feel human. Maybe it’s because we’re starting to struggle with the AI Mirror Test or we’re getting close to a successful completion of the Turing Test.
Whatever the reason, ChatGPT makes for a fascinating human-AI interaction. Use it once, though, and you’ll discover the need for improved prompt techniques to get the responses you want. Communicating with AI can be tricky, frustrating, and shockingly useful, but it can also inspire compulsive prompt-loading. You’ll see what we mean as we dive into examples of ChatGPT’s complex abilities and a few of its limitations.
ChatGPT Prompt: How Much Wood Could a Woodchuck Chuck?
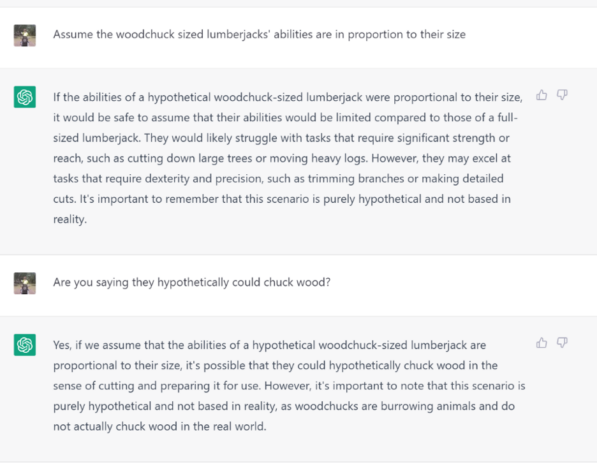
Yes, it’s a silly example. But it happens to be an amazingly challenging question for a few reasons. It requires an ability to probabilistically determine what a woodchuck is, what “chucking” wood might be, and that there may not be an actual answer.
The mind-blowing thing about this particular thread is that ChatGPT must synthesize these distinct elements into a cohesive representation of a model that it can try to answer. Even in light of the complexity of this query, ChatGPT handled it relatively well:
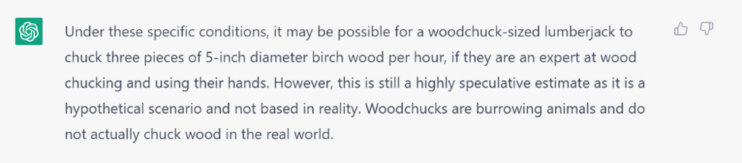
Answer: A woodchuck could, theoretically, chuck three pieces of wood per hour.
Notice how ChatGPT maintains the thread of the query throughout the conversation. In the back-and-forth, my Blackbaud colleague continued to try and nail it down to a specific answer (which seems to be a common pattern, a tit-for-tat to move from a less-certain to more-certain answer). He finally got there.
Granted, the response has quite a few caveats (such as offloading the responsibility of fact-checking on the end user), but this provides some insight into how it wrestles with questions that don’t have a clear-cut response.
ChatGPT Prompt: Symptoms and Probable Illness?
Another member of our team was ill with the symptoms described below. While he was in the doctor’s office, he queried ChatGPT to see what it predicted. And though the response (outcome of the query) was incorrect—he actually tested negative for the flu—the probabilistic answer, the most likely illness tied to the symptoms, was correct. Based on the symptoms he described and when he had the symptoms, there was a high probability that the flu was indeed the illness he was experiencing.
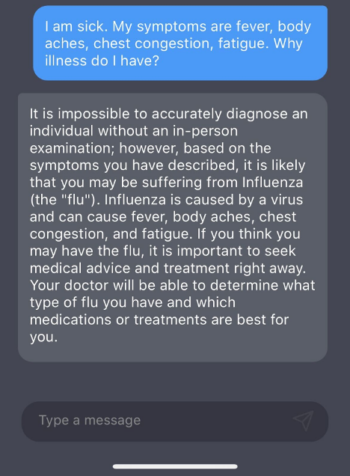
This case highlights an important fact about probability: though something may be highly probable, we must remember that there is also a probability that it is not the case. There is a chance that an alternative is the correct answer.
Be Aware of the Human Bias Toward “Resulting”
We tend to think about accuracy in terms of results, not in terms of process. Author and poker champion Annie Duke calls this “resulting.” There’s a helpful example in her book Thinking in Bets. In 2015, Pete Carroll called a passing play as the last one of Super Bowl XLIX against the Patriots. There were 26 seconds left in the fourth quarter, the Seahawks were trailing by four points, and it was second down.
The result of the play was an interception by the Patriots.
Naturally, this led to a ton of consternation about Carroll’s play-calling (“worst play-call in Super Bowl history.”) But the truth is that an interception was an extremely unlikely outcome. Out of 66 passes attempted from an opponent’s one-yard line during the season, zero had been intercepted. In the previous 15 seasons, the interception rate in that situation was about 2%.
Was Carroll wrong in calling for quarterback Russell Wilson to throw a goal-line pass? Probability was on his side. Unfortunately, victory was not. Despite the odds being in his favor, second-guessing the Seahawks continues to this day. This is a classic case of resulting.
When we work with AI (and any probabilistic approach), we need to be aware of the human bias toward resulting. We’ve seen this a ton in the Analytics space (major giving predictions, for example). Get the design or presentation of prediction solutions wrong and you can impact user trust and adoption.
ChatGPT Prompt: Upload of Sample Giving Data Without Any Query
One of our team members posted a tab-delimited dataset from our testing databases into ChatGPT’s text box, just to see what output the model would produce.
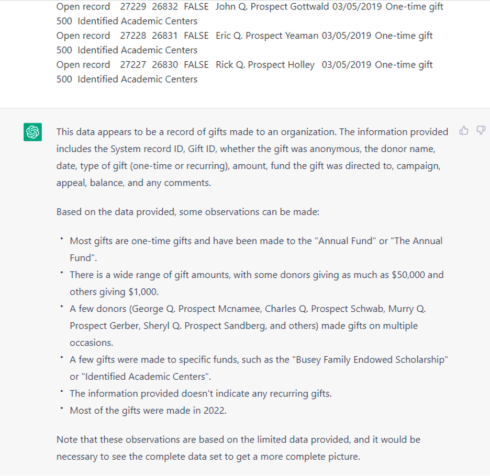
This last exhibit is particularly interesting as we think about potential applications for nonprofit organizations and the businesses that support them. From a dummy donor dataset with no context, no prompt, ChatGPT surprisingly came back with a summary of the data.
Now, not all of this is significantly meaningful, and it could be too expensive an operation for the algorithm relative to other options. But what’s fascinating is that it provided this output with no guidance. And because it’s leaning only on the data in the table, concerns about truthfulness should be less of a concern relative to other text-based questions that one might put into ChatGPT.
One note of importance here: ChatGPT takes input data (prompts) and integrates it into the broader training dataset. Essentially, it could use your data as a response to another person’s prompt. It’s key to point out again that in our experiment, we input a dummy dataset, as there could be privacy and regulatory risks associated with the use of authentic data. Already, we’re starting to see push-back due to privacy concerns in the EU: Italy temporarily has banned ChatGPT. This doesn’t mean you should shy away from the technology altogether, but you do need to be mindful of the potential risks.
Don’t Be Afraid to Experiment with Generative AI
Any emergent technology should be experimented with in many places but applied to only those where it adds (or to be probabilistic: could add) significant value. Small experiments involving novel applications over a limited time frame give us early glimmers of ideas that could be valuable and those that land flat.
As you experiment, remember:
- AI measures probabilistic outcomes (how likely is it that…)
- AI doesn’t consider “truth” in its current implementation
- Sometimes value comes from unexpected places; keep your experiments small and the learning cycles rapid
- AI will be most valuable when used to reduce uncertainty and to speed up time to action
- Where will user trust be a concern? Where might there be friction for the end user?
If you’re interested in AI-related content, check back with the ENGAGE blog often to discover how our Analytics team is experimenting with ChatGPT.
The Nonprofit CRM Built for Fundraisers
Find out how Blackbaud’s Raiser’s Edge NXT® fits your organization.
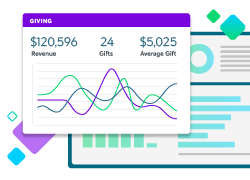