AI in Action: Deploying Responsible, Effective, and Trustworthy AI
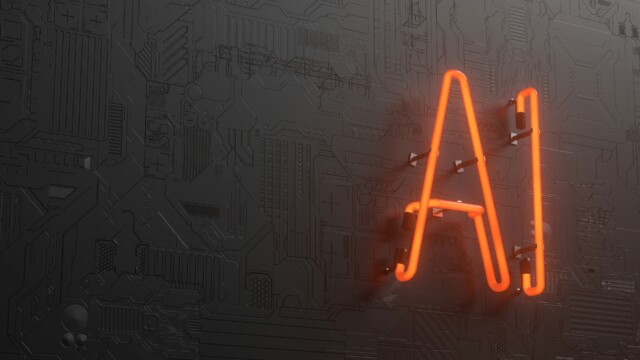
Although AI has become a buzzword recently, it’s not new. Artificial intelligence has been around since the 1950s and it has gone through periods of hype (“AI summers”) and periods with reduced interest (“AI winters”). The recent hype is driven in part by how accessible AI has become: You no longer need to be a data scientist to use AI.
With AI showing up as a wonder tool in nearly every platform we use, it’s no surprise that every industry, every business unit is suddenly racing to adopt AI. But how do you ensure the AI you want to deploy is worthy of your trust?
Responsible, effective, and trustworthy AI requires human oversight.
“At this stage, one of the barriers to widespread AI deployment is no longer the technology itself; rather, it’s a set of challenges that ironically are far more human: ethics, governance, and human values.”—Deloitte AI Institute
Understanding the Basics of AI
But human oversight requires at least a high-level understanding of how AI works. For those of us who are not data scientists, are we clear about what AI really is and what it does?
The simplest explanation I’ve seen comes from You Look Like a Thing and I Love You, by Janelle Shane. She compares AI with traditional rules-based programming, where you define exactly what should happen in a given scenario. With AI, you first define some outcome, some question you want answered. Then, you provide an algorithm with examples in the form of sample data, and you allow the algorithm to identify the best way to get to that outcome. It will do so based on patterns it finds in your sample data.
For example, let’s say you’re building a CRM to track relationships with your donors. If you plan to include search functionality, you’ll need to set up rules such as, “When a user enters a donor name in the search, return all possible matches from the CRM.” That’s rules-based programming.
Now, you might want to ask your CRM, “Which of my donors will upgrade their giving levels this year?” With AI you would first pull together examples of donors who have upgraded their giving levels in the past, tell the algorithm what you’re looking for, and it would determine which factors (if any) indicate which of your donors are likely to give more this year.
What Is Trustworthy AI?
Whether you decide to “hand over the keys” to an AI system or use it as an assistant to support the work you do, you have to trust the model. You have to trust that the training data are strong enough to lead to an accurate prediction, that the methodology for building the model is sound, and that the output is communicated in a way that you can act on. You’re also trusting that the AI was built in a responsible way, that protects data privacy and wasn’t built from a biased data set. There’s a lot to consider when building responsible AI.
Fortunately, there are several frameworks for trustworthy AI, such as those from the National Institute of Standards and Technology and the Responsible AI framework from fundraising.ai. One that we reference often comes from the European Commission, which includes seven key requirements for trustworthy AI:
- Human agency and oversight
- Technical robustness and safety
- Privacy and data governance
- Transparency
- Diversity, non-discrimination and fairness
- Societal and environmental well-being
- Accountability
These concepts aren’t new to fundraising professionals. Whether from the Association of Fundraising Professionals (AFP), the Association of Professional Researchers for Advancement (Apra), or the Association of Advancement Services Professionals (AASP), you’ll find overlap with fundraising ethics statements and the guidelines for trustworthy AI. Technology is always changing, but the guiding principles should stay the same.
Human Agency and Oversight: Decision-making
While each component of trustworthy AI is crucial, for this post we’re focused on the “human agency and oversight” aspect. The European Commission explains this component as follows:
“AI systems should empower human beings, allowing them to make informed decisions and fostering their fundamental rights. At the same time, proper oversight mechanisms need to be ensured, which can be achieved through human-in-the-loop, human-on-the-loop, and human-in-command approaches.”
The concept of human agency and oversight is directly related to decision-making. There are decisions to be made when building the models, decisions when using the models, and the decision of whether to use AI at all. AI is another tool in your toolbox. In complex and nuanced industries, it should complement the work done by subject matter experts (not replace them).
Decisions When Building the Models
When building a predictive AI model, you’ll have many questions. Some examples:
- What should you include in your training data?
- What outcome are you trying to predict?
- Should you optimize for precision or recall?
All predictions are going to be wrong some percentage of the time. Knowing that, you’ll want to decide whether it’s better to have false positives or false negatives (People and AI Research from Google provides a guidebook to help with these types of decisions). At Blackbaud, we had to decide whether to optimize for false negatives or false positives while building our new AI-driven solution, Prospect Insights Pro. Prospect Insights Pro uses artificial intelligence to help fundraisers identify their best major gift prospects.
- Our false negative: A scenario where the model does not predict a prospect will give a major donation, but they would have if asked
- Our false positive: A scenario where the model predicts a prospect will give a major donation if asked, but they do not
Which scenario is preferred? We found the answer to this question could change based on whether you have an AI system working on its own or alongside a subject matter expert. If you keep a human in the loop, then false positives are more acceptable. That’s because a prospect development professional can use their expertise to disqualify certain prospects. The AI model will prioritize prospects to review based on patterns it identifies in the data, and then the subject matter expert makes the final decision on what action to take based on the data and their own expertise.
Decisions When Using the Model
When deploying an AI model, or using one from a vendor, you’ll have more questions to consider. Examples include:
- What action should I take based on the data?
- How does the prediction impact our strategy?
To make these decisions when working with AI, you must keep a human in the loop.
Leah Payne, Director of Prospect Management and Research at Longwood University, is head of the team that participated in an early adopter program for Prospect Insights Pro. As the subject matter expert, she makes the decision on whether to qualify identified prospects, as well as which fundraiser to assign each prospect to once they are qualified. Prospect Insights Pro helped Payne find a prospect who wasn’t previously on her radar.
“It makes the process of adding and removing prospects to portfolios much more efficient because I can easily identify those we may have missed and remove low likelihood prospects to support portfolio churn,” she said.
For this newly surfaced prospect, it was Payne, not AI, making the final call. Payne decided to assign the prospect to a specific fundraiser because she knew they had shared interests. Using the data to inform her qualification and assignment decisions, Payne was able to get to those decisions faster by working with AI. But she brought a level of insight that AI alone would have missed.
When to Use AI
Prediction Machines identifies scenarios where predictive AI can work really well. You need two elements:
- A rich dataset for an algorithm to learn from
- A clear question to predict (the narrower and more specific the better)
But that framework still focuses on the question of can we use AI. We also need to consider whether we should use AI. To answer, consider the following:
- Potential costs
- Potential benefits
- Potential risks
Evaluating potential risks for your AI use case can help determine the importance of keeping a human in the loop. If the risk is low, such as Spotify predicting which song you’ll like, then you may be comfortable with AI running on its own. If the risk is high, then you’ll want to keep a human in the loop, as they can mitigate some risks (but not all of them). For example, Payne stresses that due diligence remains essential when evaluating potential donors. Someone may look great on paper, but their values may not be aligned with the values of your organization.
The Value of Relationships
Fundraising is about building relationships, not building models. If you let the machines do what they do best—finding patterns in large amounts of data—that frees up humans to do what they do best, which is forming authentic connections and building strong relationships.
Payne’s colleague at Longwood University, Director of Donor Impact Drew Hudson, said no algorithm can beat the old-time art of chitchatting.
“Data mining exercises can inaccurately assess capacity and no AI drill is going be able to identify a donor’s affinity accurately,” he said.
AI can help you save time, but AI cannot form an authentic connection with a potential donor.