AI, Analytics, and Your Nonprofit’s Technology Strategy
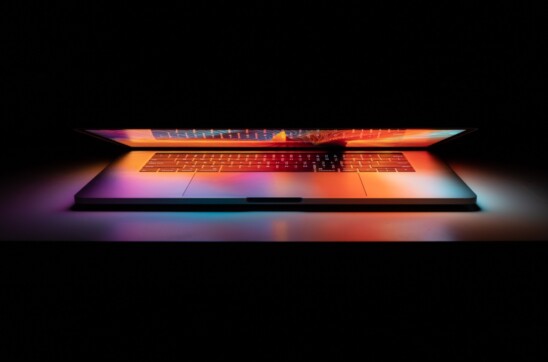
Nonprofit leaders often have an all-or-nothing response to the question of using artificial intelligence (AI) tools. Either they’re all in from the start—“What if AI solves all our problems?”—or they opt out before they even understand what AI could do for them.
In most cases, AI will land somewhere in between solving everything and just not being the perfect tool for you. But you need to be open-minded about AI, recognizing that while it is a powerful (and, to me, very cool) innovation, it isn’t always going to be the right fit.
To help understand where AI can or should play a role, I would like to walk you through a methodology we use at Blackbaud, called the Outcomes-Based Framework. We use it to assess whether predictions and AI may play a role in solving a particular problem. We’ll look at examples, but note that they reference predictive intelligence, meaning they use machine learning models fed with data to predict something.
This is separate from generative AI, also a powerful (and, to me, very cool) innovation. But generative AI tools like chatGPT have different use cases from the predictive intelligence we’re exploring here as a component of your nonprofit’s technology strategy.
How Will AI Help Your Team? Chart Your Course webinars available on demand
To get started, it helps to have in mind a problem you think might be solved by AI. Maybe you have a particular type of fundraising that just doesn’t perform the way you expect. Are there campaigns you think would make a huge difference, but the up-front costs mean you need to be sure before you commit?
Or maybe it’s all still so new to you, that you can’t even venture a guess about what problem AI might solve for your nonprofit organization. That’s okay, too. Uncertainty is exactly what AI aims to solve.
As we work through use cases, keep the questions coming:
- What outcome are you hoping to drive?
- What is the uncertainty you hope to solve?
- What are the implications if the prediction is correct? If it’s not?
A Brief History of AI
Computer science, artificial intelligence, neuroscience, and cognitive science all began as part of the same field centered around the exploration of thought. Each of these disciplines seeks an understanding of what it is to think and learn.
Predictive analytics, AI, and machine learning remained strictly academic from the 1940s until the 1980s, when computer power started to become available for commercial applications. But that does not mean its concepts were not being applied.
The Vietnam War and Big Data: A Case Study in Machine Learning
Robert S. McNamara was many things: officer in the Army Air Force during WWII, president of Ford Motor Co., president of the World Bank. It was his work as Secretary of Defense during the Vietnam War, though, that connects him to what we now call data science and big data analytics.
Leveraging techniques he and his “Whiz Kids” first practiced in the Pacific Theater of WWII, McNamara devised a data-lead strategy for the commitment of American troops and materiel in Vietnam. The “McNamara Line” was a series of electronic monitors, sensors, and human intelligence mixed with real-time reports to make predictions. These predictions, based on assumptions about the North Vietnamese army and existing American warfighting heuristics, were used to determine what the best military option might be.
To a certain extent, this could sound like any major corporation today—heavy data use, best practices, and smart people combining to set strategy. But McNamara and his group were over-reliant on data, particularly data related to outcomes they preferred, often dropping negative data or data that did not fit the model completely.
How to Spot Responsible AI: Three Questions to Start With—Free download
Take a look at the questions posed earlier, first related to McNamara’s goals and then to your own.
What outcome was Robert McNamara hoping to drive?
- At a high-level, winning the Vietnam War
What is the key uncertainty he hoped to solve?
- The best use of American resources
- The predicted next decisions of the North Vietnamese
What were the implications of the prediction(s) if they had been correct?
- The implication is that if the McNamara Line had driven good strategy, the United States would have had a near-perfect counterstrategy to their adversary. With hindsight, this probably should have been a warning sign of potential overreliance on data.
H2. How Does This Apply to AI in the Social Good Space?
It’s kind of weird to imagine the connection between McNamara’s big data in Vietnam to a tech strategy for a nonprofit. So, what does this all mean for you? Fair question.
Let’s use that Outcomes-Based Framework mentioned at the beginning and work through a sample problem. The framework we use at Blackbaud is an adjusted version of the AI Canvas, an easier introduction to the concept derived from the book, Prediction Machines.
For this exercise let’s imagine a scenario: You are a fundraiser at a good-sized charity. You and your team are pretty successful, but you have noticed that some supporters donate one time and never engage again. Others eventually convert to become sustaining donors. You would like to know what makes one person more likely to convert over another.
Before you can figure out if AI is right for your use case, how would you even use AI on your data? If you’re a Blackbaud customer, you have an array of tools and services available that are tailored to your needs, such as Prospect Insights. Third-party businesses also offer AI-as-a-service, where you send them your data, maybe through an API or a file transfer, and they return predictions to you.
Now let’s determine if AI could bring clarity to your problem.
What outcome are you hoping to drive?
- Identify one-time donors likely to convert to larger gifts.
- Make clear parameters: What does “larger” mean to you in this context? Do donors just give a second time, commit to routinely give, donate 150% more than the initial gift?
- Tangible and measurable parameters are important for leveraging AI. Otherwise, you can end up with too broad a prediction (which would be unhelpful) or too narrow (which leaves a lot of potential gifts on the table).
What single task or decision do you want to analyze that plays an important role in the stated outcome?
- In this case we are talking about conversions.
- As you may have gathered from the previous question, we need to define what a conversion means in this scenario. Just any additional gift after the first one? An agreement to a series of gifts? Either outcome is good, but it’s likely they each would require a different approach, a different level of communication. With limited marketing resources, you probably want your prediction to tell you who to contact and what to ask for.
What measure of performance do you want to use? In other words, how will you know you’ve nailed it?
- This is your goal and it is almost always a number. Do you want a 3% increase in conversions? A 10% increase? What is your cutoff point to determine if this effort was a success or failure?
What do you do once you have this prediction?
- You’ve turned your trained AI models loose on a CRM full of potential converters. It has predicted Ian as a likely candidate. It has told you what kind of gift I am likely to give. Now what? Will you call me, email me, send me a handwritten note?
All the AI and machine learning in the world isn’t worth anything if you don’t know what to do with it. In our example, you would use AI’s predictive capabilities to create an action plan for building your sustaining donor program.
One blog isn’t going to turn you into an AI expert. But hopefully it will help you to ask the right questions and point you to the many benefits of working with a software partner offering deep expertise in both AI technology and in the social good space. Learn more about Intelligence for Good® and explore how nonprofit organizations like yours are tapping the most accessible, powerful, and trusted AI and analytics tools to modernize their technology strategy.
Free Webinar
Artificial Intelligence: Analytics and Your Technology Strategy—Finding the Right Fit